4EU+ SUMMER SCHOOL ON ARTIFICIAL INTELLIGENCE
Gargnano del Garda, Italy
15-19 August 2022
Photos
Info
The school will present recent advances on various aspect of science
and technology of artificial intelligence and machine learning,
including theory, techniques, methodologies, and applications.
The Summer School is part of the activities of the 4EU+ Flagship 3:
"Transforming science and society: Advancing information, computation and communication".
Questions related to the Summer School should be sent to Prof. Vincenzo Piuri (vincenzo.piuri@unimi.it)
Venue
Feltrinelli Palace, Gargnano del Garda, Italy, 15-19 August 2022
[Info]
Accomodation
The University of Milan will book reasonably priced rooms for
participants in one of the following locations in Gargnano del Garda:
- Palazzo Feltrinelli, via Castello 4 (location of the educational activities),
- Palazzo Bertolini, piazza Feltrinelli 4 (a building near Palazzo
Feltrinelli).
Target Audience
The school is directed to PhD students in computer science and
engineering, automation engineering, biomedical engineering,
mathematics, statistics, physics, and other scientific and
technological sectors.
Application
In order to apply please contact your local 4EU+ Flagship 3
Coordinator. The number of attendees in physical presence is limited.
A limited number of additional attendees will also be allowed remotely.
Attendance is restricted to students of the Universities of the 4EU+ Alliance.
A certificate of attendance will be given to all participants. The
school will also constitute a PhD course of the University of Milan,
issuing - if a final exam will be passed - a certificate of
competences with ECTS to PhD students.
Workshop
In some of the evenings, the participants will be encouraged to make a
short presentation of their research areas and achievements and
interact with the lecturers. Participants can also submit a paper on
his/her research topic to be published in the peer-reviewed
proceedings of the Workshop.
Mobility program
The 4EU+ mobility program will support travel of participants to
attend the School. For more information about this travel support,
please ask your local 4EU+ Flagship 3 Coordinator. Accomodation and
meals at the School are offered by the 4EU+ mobility program.
Meals
All meals (breakfast, coffee break, lunch, dinner) will be served at Feltrinelli Palace, near the area designated for the lectures.
Remote Attendance
You can join the educational activities by using Zoom at
[Link].
4EU+ Flagship 3 Coordinators
University of Warsaw
Błażej Miasojedow
b.miasojedow@uw.edu.pl
|
Heidelberg University
Theresa Möke
theresa.moeke@iwr.uni-heidelberg.de
|
Charles Univeristy
Jan Kratochvil
honza@kam.mff.cuni.cz
|
University of Milan
Vincenzo Piuri
vincenzo.piuri@unimi.it
|
University of Copenhagen
Katja Sander Johannsen
kjo@science.ku.dk
|
Sorbonna
Gérard Biau
gerard.biau@sorbonne-universite.fr
|
Speakers
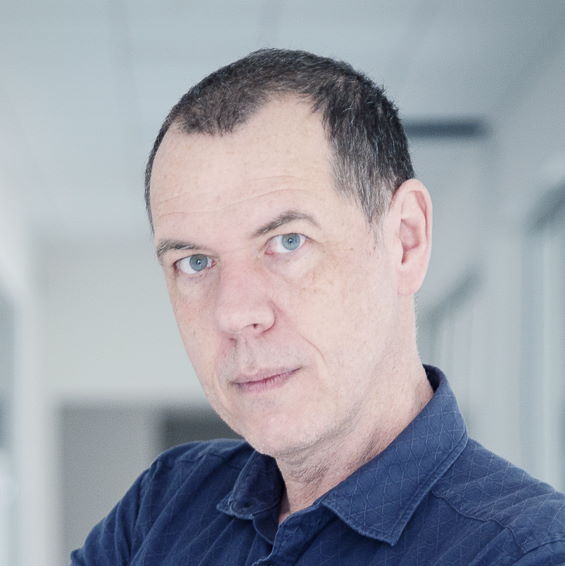
Roman Neruda
Czech Academy of Sciences, Czech Republic
Automated machine learning and neural architecture search
|
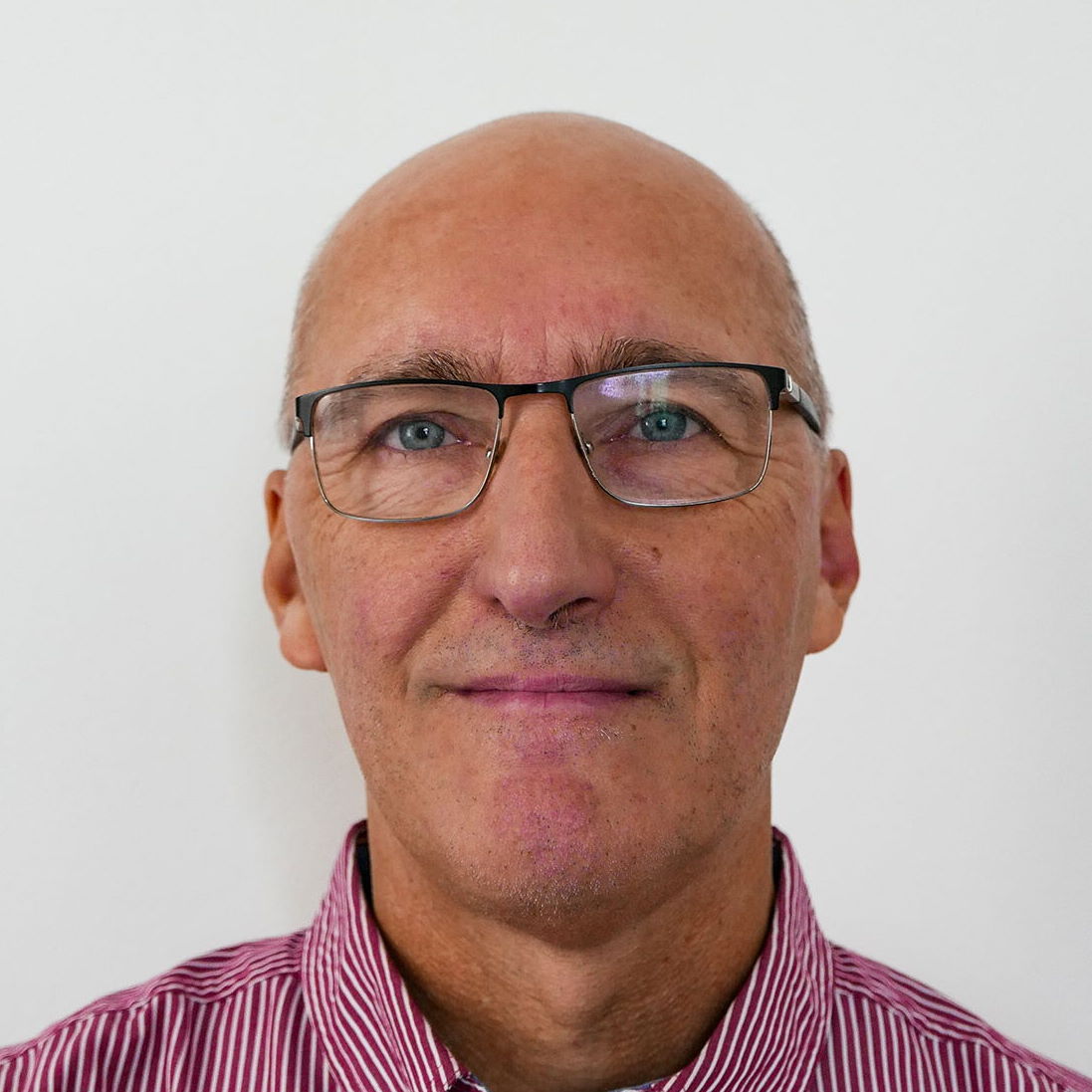
Ondřej Čepek
Charles University, Czech Republic
Knowledge compilation: An overview
|
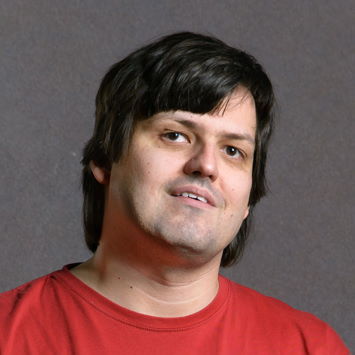
Martin Pilát
Charles University, Czech Republic
Surrogate models in evolutionary algorithms
|
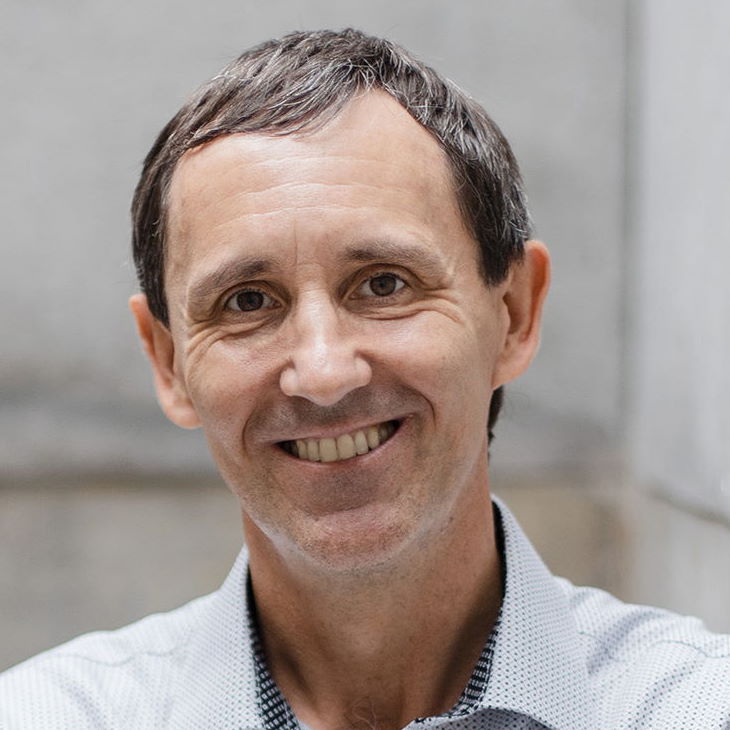
Roman Barták
Charles University, Czech Republic
Multi-agent path finding: From algorithms to applications
|
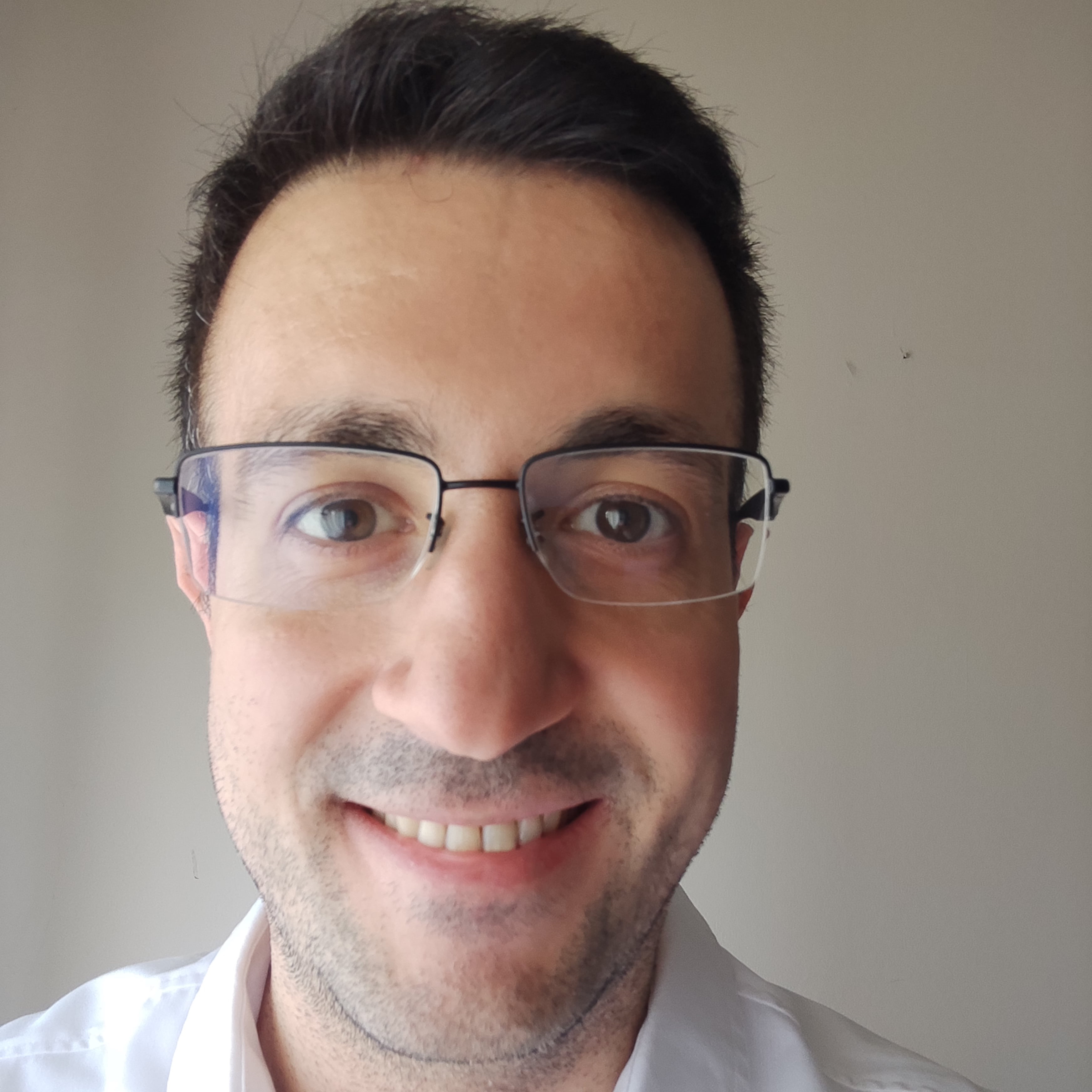
Pasquale Coscia
Università degli Studi di Milano, Italy
Predictive modelling in urban contexts
|
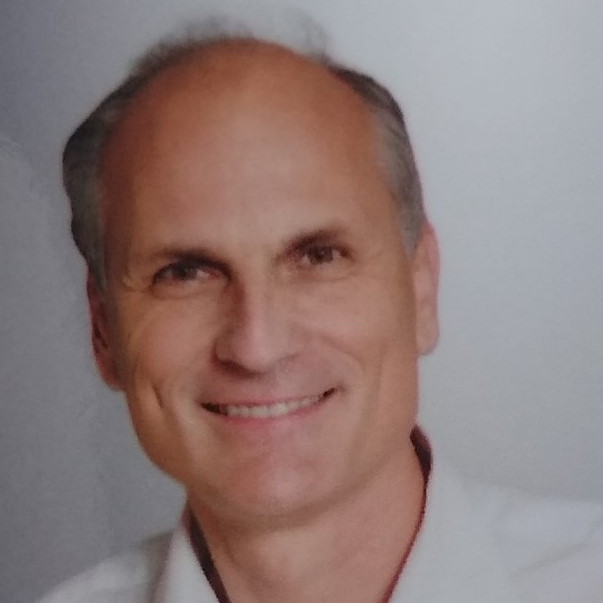
Jürgen Hesser
Heidelberg University, Germany
Causal Inference - assessing the individual treatment effect
|
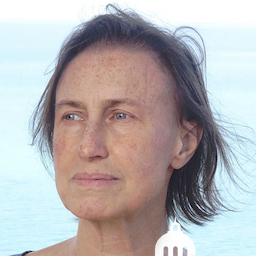
Isabelle Bloch
Sorbonne University, France
Hybrid AI for knowledge representation and model-based medical image understanding - Towards explainability
|
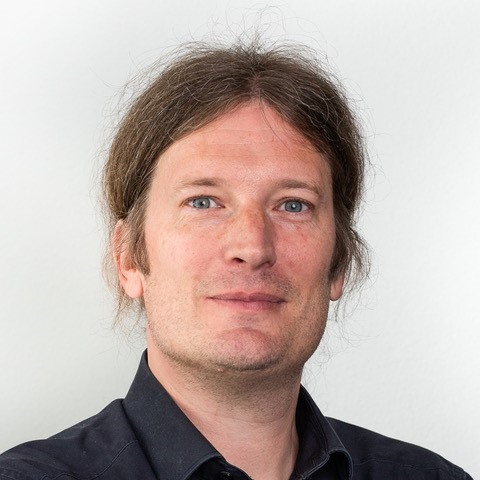
Christian Igel
University of Copenhagen, Denmark
1, 2, 3: Semantic segmentation with fully convolutional neural networks
|

Simon Weissmann
Universität Heidelberg, Germany
Introduction to Bayesian inference and filtering
|

Iveta Mrazova
Charles University, Czech Republic
Social networks and their analysis
|
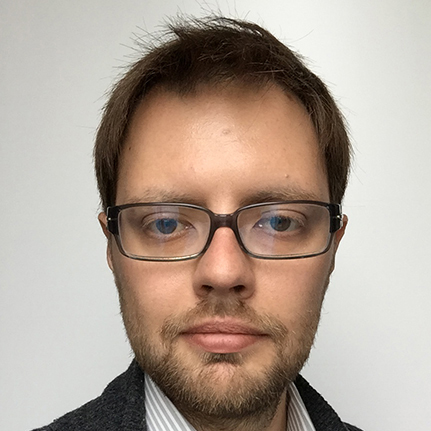
Angelo Genovese
Università degli Studi di Milano, Italy
GANs for synthetic data generation: Application to data anonymization
|
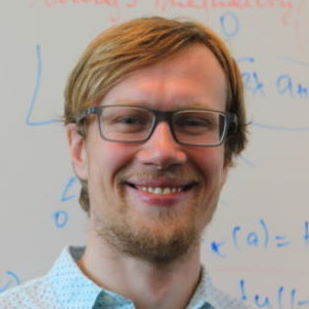
Jacek Cyranka
University of Warsaw, Poland
Foundations of interpretable and reliable reinforcement learning
|
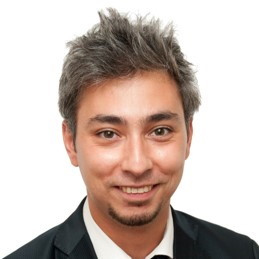
Massimo Rivolta
Università degli Studi di Milano, Italy
The importance of Explainable AI in the context of biomedical data
|
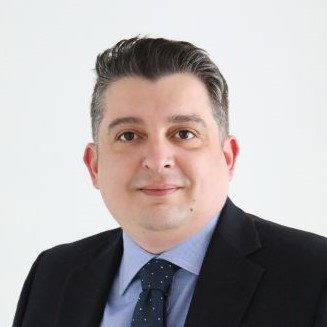
Ruggero Donida Labati
Università degli Studi di Milano, Italy
Artificial Intelligence for biometric technologies, systems and applications
|
Schedule
|
17 August 2022
|
18 August 2022
|
08:15 – 09.15
|
Breakfast *
|
Breakfast *
|
09:30 – 11.00
|
Ruggero Donida Labati
Università degli Studi di Milano, Italy
Artificial Intelligence for biometric technologies, systems and applications
|
Simon Weissmann
Universität Heidelberg, Germany
Introduction to Bayesian inference and filtering
|
11.00 – 11.30
|
Coffee break *
|
Coffee break *
|
11:30 – 13.00
|
Ruggero Donida Labati
Università degli Studi di Milano, Italy
Artificial Intelligence for biometric technologies, systems and applications (cont'd)
|
Simon Weissmann
Universität Heidelberg, Germany
Introduction to Bayesian inference and filtering (cont'd)
|
13.00 – 14.00
|
Lunch *
|
Lunch *
|
14.00 – 15.30
|
Isabelle Bloch
Sorbonne University, France
Hybrid AI for knowledge representation and model-based medical image understanding - Towards explainability
|
Iveta Mrazova
Charles University, Czech Republic
Social networks and their analysis
|
15.30 – 16.00
|
Coffee break *
|
Coffee break *
|
16.00 – 17.30
|
Christian Igel
University of Copenhagen, Denmark
1, 2, 3: Semantic segmentation with fully convolutional neural networks
|
Angelo Genovese
Università degli Studi di Milano, Italy
GANs for synthetic data generation: Application to data anonymization (Part A)
|
18.00 – 19.30
|
Workshop
|
Workshop
|
19.30
|
Dinner *
|
Dinner *
|
Abstracts
|
Roman Neruda
Czech Academy of Sciences, Czech Republic
Automated machine learning and neural architecture search
|
Abstract: Automated machine learning (AutoML) is a process of automating
the design of machine learning models. It can be applied to create pipelines of traditional
ML models and ensembles, or to search for neural network architectures (NAS). In the talk
we will present an overview of the three main components of AutoML - the search spaces
representing ML models, the search strategies, and performance estimation of the models.
|
Bio: Roman Neruda is a researcher at the Institute of Computer Science of the
Czech Academy of Sciences (ICS CAS), and a lecturer at the Faculty of Mathematics and Physics,
Charles University in Prague. His research interests include automated machine learning, meta-learning,
evolutionary computing and adversarial examples.
|
Ondřej Čepek
Charles University, Czech Republic
Knowledge compilation: An overview
|
|
Abstract: In this talk we survey several types of knowledge representation languages
(Boolean formulas, binary decision diagrams, list-based representations, Boolean circuits, and
negational normal forms) and compilation among these languages. The talk is based mainly on the Knowledge
Compilation Map framework [Darwiche and Marquis, 2002] and several recent extensions of this framework.
|
Bio: Ondřej Čepek is an associate professor of theoretical computer science at Charles
University in Prague (Czech Republic). He obtained his Ph.D. at Rutgers University (USA) in 1995. His
research interests include knowledge representation languages, theory of Boolean functions, complexity
theory, and design and analysis of algorithms.
|
|
Martin Pilát
Charles University, Czech Republic
Surrogate models in evolutionary algorithms
|
Abstract: Evolutionary algorithms are strong optimizers.
However, one of their main disadvantages is the large number of objective
function evaluations they need to find a solution. One of the possible ways
to reduce this number is to use surrogate models - fast approximations of
the objective function, typically based on regression models. In the talk,
we will discuss, how surrogate models are constructed and used in evolutionary
algorithms including in cases where the individual encoding is complicated.
|
Bio: Martin Pilát is an assistant professor at Charles University,
Faculty of Mathematics and Physics. His has long experience applying surrogate models
in evolutionary algorithm for problems with complex fitness functions including multi-objective
ones. His research interests are evolutionary algorithms, machine learning, and their applications.
|
Roman Barták
Charles University, Czech Republic
Multi-agent path finding: From algorithms to applications
|
|
Abstract: Multi-agent Path Finding (MAPF) deals find finding collision-free paths
for a group of agents (robots) moving in a shared environment, such as, warehouses,
traffic junctions, airports, and computer games. The tutorial will introduce the
MAPF problem and its variants, describe the core techniques to solve it, both reduction-based
and search-based, and overview some existing and possible future applications.
|
Bio: Roman Barták is a full professor at Charles University, Faculty of Mathematics and Physics.
He coordinates undergraduate, graduate, and postgraduate AI programs there. His main research
area is automated planning and scheduling with the ultimate research goal to design autonomous
agents (e.g., robots) that can plan their activities, do decisions, and act in real environment.
Roman Barták is AAAI and ACM Senior Member, and EurAI Fellow.
|
|
Pasquale Coscia
Università degli Studi di Milano, Italy
Predictive modelling in urban contexts
|
Abstract: Mimicking human ability to forecast future events is essential for
developing social-aware robots, self-driving cars and advanced video-surveillance applications.
Despite recent advancements in machine vision and temporal sequences modelling, understanding how
humans interact with the surrounding environment still remains a challenging task. This talk will
present the main used evaluation protocols and datasets for predicting future human positions in
crowded environments, and will discuss the main aspects involved in this task (e.g., human-human
and human-scene interactions). Then, a number of recently proposed approaches for predicting pedestrian
positions as well as intentions will we presented, and multiple techniques to condition future dynamics analyzed.
|
Bio: Pasquale Coscia is an Assistant Professor (RTDa) at the Department of
Computer Science of University of Milan (Italy) and member of the Industrial, Environmental and
Biometric Informatics (IEBI) Laboratory. He received both his M.Sc. degree in Computer Engineering
and Ph.D. degree in Industrial and Information Engineering from University of Campania (Italy)
in 2014 and 2019, respectively. From 2019 to 2022, he was a post-doctoral researcher at University of
Padova (Italy). In 2016, he was involved in the Visiting Research Program (VRP) at the NATO STO-CMRE in
La Spezia (Italy). He also co-organized the Benchmarking Trajectory Forecasting Models (BTFM) workshop
series at ECCV'20 and ICCV'21. His main research interests are in the areas of computer vision,
neural networks and machine learning.
|
Jürgen Hesser
Heidelberg University, Germany
Causal Inference - assessing the individual treatment effect
|
|
Abstract: The concept of causal inference is discussed in this lecture part by
the example of an individual treatment effect.
Let us assume that we have observational data. Patients that can be
characterized by clinical patient records are to be treated with
either of two different treatments. Can we determine for each
treated patient what would have been the treatment outcome if he or
she received the other treatment?
Now, observational data has to fulfill some criteria to have a
chance for this prediction, which are the absence of hidden
confounders influencing the outcome, the non-vanishing probability
to receive each of the two treatments, and the consistency where
patients with the same clinical records experience the same outcome
for a given treatment.
The presentation gives an overview of this field and potential and
limitations of current methods.
|
Bio: Prof. Dr. Jürgen Hesser is Professor at the Heidelberg University. He
has been deputy professor at the University of Mannheim and guest
lecturer at the Harvard University. He received his PhD degree in
physics at the Heidelberg University in 1992.
His main research interests are in data analysis and modeling with
specific focus on medicine. Main topics are inverse problems for
image reconstruction, restauration, and analysis, simulation, and
modeling as well as instrumentation. Results have been published in
more than 150 papers in international journals, books, and conference
publications.
|
|
Isabelle Bloch
Sorbonne University, France
Hybrid AI for knowledge representation and model-based medical image understanding - Towards explainability
|
Abstract: This presentation will focus on hybrid AI, as a step towards explainability,
more specifically in the domain of spatial reasoning and image understanding. Image understanding benefits
from the modeling of knowledge about both the scene observed and the objects it contains as well as their
relationships. We show in this context the contribution of hybrid artificial intelligence, combining different
types of formalisms and methods, and combining knowledge with data. Knowledge representation may rely on
symbolic and qualitative approaches, as well as semi-qualitative ones to account for their imprecision or
vagueness. Structural information can be modeled in several formalisms, such as graphs, ontologies, logical knowledge
bases, or neural networks, on which reasoning will be based. Image understanding is then expressed as a problem of
spatial reasoning. These approaches will be illustrated with examples in medical imaging, illustrating the usefulness of
combining several approaches.
|
Bio: Isabelle Bloch graduated from the Ecole des Mines de Paris, Paris, France, in 1986,
and received the MSc degree from the University Paris 12, Paris, in 1987, the PhD degree from the Ecole Nationale
Supérieure des Télécommunications (Télécom Paris), Paris, in 1990, and the Habilitation
degree from University Paris 5, Paris, in 1995. She has been a Professor at Télécom Paris until 2020 and
is now a Professor at Sorbonne Université. Her current research interests include symbolic and hybrid artificial
intelligence, explainability, 3D image understanding, mathematical morphology, information fusion, fuzzy set
theory, structural, graph-based, and knowledge-based object recognition, spatial reasoning, and medical imaging.
|
Christian Igel
University of Copenhagen, Denmark
1, 2, 3: Semantic segmentation with fully convolutional neural networks
|
|
Abstract: U-Nets are fully convolutional neural networks
known for their excellent performance in image segmentation.
We will discuss U-Nets for semantic segmentation of 1-, 2- and 3-dimensional
data with application examples from remote sensing and medical data analysis.
|
Bio: Christian Igel is a professor at DIKU, the Department of Computer Science
at the University of Copenhagen (UCPH). He studied Computer Science at the Technical University
of Dortmund, Germany. In 2002, he received his Doctoral degree from the Faculty of Technology,
Bielefeld University, Germany, and in 2010 his Habilitation degree from the Department of
Electrical Engineering and Information Sciences, Ruhr-University Bochum (RUB), Germany.
From 2003 to 2010, he was a Juniorprofessor f at the Institute for Neural Computation, RUB.
In October 2010, He was appointed professor with special duties in machine learning at DIKU.
Since December 2014 he is a full professor at DIKU.
Christian is the director of the SCIENCE AI Centre at UCPH and a co-lead of the Pioneer Centre
for Artificial Intelligence, Denmark.
He is a Fellow of ELLIS, European Lab for Learning and Intelligent System. Among others,
he serves as an Editor of the German Journal on Artificial Intelligence (KI) and an Associate
Editor of the Evolutionary Computation Journal (ECJ) and the Artificial Intelligence Journal (AIJ).
|
|
Simon Weissmann
Heidelberg Universität, Germany
Introduction to Bayesian inference and filtering
|
Abstract: In data assimilation we deal with the combination of two sources of information:
a time-dependent physical model describing the hidden signal of interest and the observation model describing
the corresponding measurements. After a brief introduction into the field of Bayesian inverse problems, we
discuss the application to the Bayesian filtering problem. The task is to sequentially update the filtering
distribution when accessing new data through measurements. This course will give an overview of commonly
applied filtering methods such as the (ensemble) Kalman filter, extended Kalman filter and particle filters.
|
Bio: Simon Weissmann is a postdoctoral researcher at the University of Heidelberg and a member
of the Interdisciplinary Center for Scientific Computing (IWR) in the work group Machine Learning. He received
his PhD on sampling and optimization methods for inverse problems at the University of Mannheim as a member of
the research training group Statistical Modeling of Complex Systems and Processes. His research interest lies
at the interface between probability theory, optimization and numerical analysis. In particular, his research
focuses on particle based methods for solving (Bayesian) inverse problems.
|
Iveta Mrazova
Charles University, Czech Republic
Social networks and their analysis
|
|
Abstract: Social networks emerging in today's interlinked world are rich in structure,
and their analysis has raised new conceptual opportunities. Usually, we understand social networks as
graphs. Their nodes correspond to individuals, and edges represent their mutual relationships. In this
lecture, we will introduce fundamental principles governing the structure of social networks. In particular,
we will specify various measures indicating the significance of the involved social actors (centrality measures)
and discuss tools available for social network analysis. These will include, e.g., the models and algorithms
applicable to community discovery, influence analysis, and link prediction. The field's newer topics represent
dynamic social networks and the so-called graph neural networks. The talk illustrates the discussed models and
techniques on both artificial and real-world examples of social networks.
|
Bio: Iveta Mrazova is Associate Professor at Charles University, Czech Republic.
She graduated from F. Schiller University in Jena, Germany, in 1989 and received her Ph.D. from the
Institute of Computer Science of the Czech Academy of Sciences in Prague in 1997. In 2002/3, she was a
Fulbright fellow at Missouri University of Science and Technology in Rolla, USA. Since 2014, she has
served for eight years as the Head of the Department of Theoretical Computer Science and Mathematical
Logic at the Faculty of Mathematics and Physics, Charles University. Her research interests include
artificial intelligence, machine learning, data mining, social network analytics, and knowledge extraction.
|
|
Angelo Genovese
Università degli Studi di Milano, Italy
GANs for synthetic data generation: Application to data anonymization
|
Abstract: Generative Adversarial Networks (GAN) have recently become the de-facto standard for generating
highly realistic synthetic signal and images, which in most cases a human operator cannot distinguish from real samples.
The capability of GAN to generate realistic content is finding important applications in the biometrics field, for example
to generate synthetic yet highly realistic biometric traits, that are not associated with any real individual and therefore
do not pose privacy issues. In this talk, we will describe how GANs work and how they can be used to generate synthetic biometric
information. We will show that many face pictures present on the web contain iris with a resolution sufficient to perform a
biometric recognition, and how a GAN-based approach can be used to anonymize such images by replacing the iris with a synthetically
generated one.
|
Bio: Angelo Genovese (S'12-M'15) received the Ph.D. degree in computer science from the Università degli Studi
di Milano, Italy, in 2014. He has been an Assistant Professor in Computer Science with the Università degli Studi di Milano since
2019. He has been a Visiting Researcher with the University of Toronto, ON, Canada. Original results have been published in over
60 papers in international journals, proceedings of international conferences, books, and book chapters. His current research
interests include signal and image processing, three-dimensional reconstruction, artificial intelligence for medical imaging,
industrial and environmental monitoring systems, biometric systems, and design methodologies and algorithms for self-adapting
systems. Dr. Genovese is an Associate Editor of the Journal of Ambient Intelligence and Humanized Computing (Springer) and Array (Elsevier).
|
Jacek Cyranka
Warsaw University, Poland
Foundations of interpretable and reliable reinforcement learning
|
|
Abstract: The idea of the talk is to showcase my recent research interests,
concentrated on research topic related to study of interpretable and reliable
reinforcement learning (RL) algorithms with applications to robotics and space missions.
I will give a brief introduction to the state of the art of off-policy RL methods applied
to problems arising in robotics studied in both online and offline setups.
Then, based on recent work in progress, I will discuss about improvements that can be achieved
using so-called state-planning policies, and development
of new challenging environments dedicated for continuous RL. Finally, I will demonstrate using
a simple case-study that the AI controllers may not always
be robust.
|
Bio: Jacek Cyranka is an assistant professor in Computer Science at
the Faculty of Mathematics, Informatics and Mechanics, the University of Warsaw,
where he leads an interdisciplinary research group focusing on the general topic of
reliable AI, model-based reinforcement learning, and validated
optimal control design for continuous robotic tasks. He received his PhD from Jagiellonian
University in 2013 and prior to joining the University of Warsaw,
he was a postdoc at Rutgers University and University California, San Diego.
|
|
Massimo Rivolta
Università degli Studi di Milano, Italy
The importance of Explainable AI in the context of biomedical data
|
Abstract: The difficulty of deploying AI models within the clinical practice is
related to a multitude of factors. Data scarcity, trust in the AI model and
data privacy are among the most common ones. In this lecture, we will
explore the reasons for the importance of Explainable AI in the context of
biomedical data. In particular, open problems will be discussed, techniques
will be analyzed and attempts to tackle some aspects will be described.
|
Bio: Dr. Massimo Walter Rivolta is an assistant professor and member of the
Biomedical image and Signal Processing Group at the University of Milan,
Italy. He received the M.Sc. degree in Biomedical Engineering from the
Politecnico di Milano, Italy, and the Ph.D degree in Computer Science from
the Università degli Studi di Milano, Italy.
His research interests include signal processing, feature extraction,
computational intelligence and computerized simulations for biomedical
applications. Specifically, he aims to create automatic and innovative tools
for processing diverse types of biomedical data such as electrocardiographic
signals, heart-rate variability series, and accelerometer signals.
|
Ruggero Donida Labati
Università degli Studi di Milano, Italy
Artificial Intelligence for biometric technologies, systems and applications
|
|
Abstract: The number of biometric applications and devices is continuously growing
on a global scale and biometrics is pervasively entering the everyday life of users. This relevant
expansion is producing new challenges and requirements to be fulfilled by the designers. Features
such as adaptability, enhanced interactions with the user, robustness to non-ideal conditions,
real-time capability, privacy-compliance, and high accuracy are strongly required in innovative
applications and solutions, such as: cyber security, smart devices, and ambient intelligent infrastructures.
The course will focus on innovative biometric recognition approaches and systems, with specific attention
on recent approaches based on artificial intelligence and deep learning techniques. Artificial intelligence
methods and, in particular, Deep learning approaches, are capable to learn discriminative features directly
from complex multidimensional signals and increase the accuracy, adaptability, and robustness to non-ideal
conditions of biometric systems with respect to traditional approaches. The course will present biometric
systems from a technological point of view and will provide an excursus of recent artificial intelligence
approaches, including deep learning methods with current strong points and limitations.
|
Bio: Ruggero Donida Labati received the Ph.D. degree in computer science from the Università degli
Studi di Milano, Italy, in 2013. Since April 2022, he has been an Associate Professor with the Università degli Studi
di Milano. He has been a Visiting Researcher with Michigan State University, MI, USA. His original results have
been published in more than 70 papers in international journals, proceedings of international conferences, books,
and book chapters. His research interests include biometric systems, artificial intelligence and machine learning,
signal and image processing, pattern analysis and recognition, and theory and industrial applications of neural
networks. Dr. Donida Labati is currently an Associate Editor of Journal of Ambient Intelligence and Humanized Computing (Springer).
|